top of page

mmWave/THz ISAC
1. Radio SLAM

5G millimeter wave (mmWave) signals can enable accurate positioning in vehicular networks when the base station (BS) and vehicles are equipped with large antenna arrays. However, radio-based positioning suffers from multipath signals generated by different types of objects in the physical environment. Multipath can be turned into a benefit, by building up a radio map (comprising the number of objects, object type, and object state) and using this map to exploit all available signal paths for positioning. Mapping and positioning using 5G can be categorized as a simultaneous localization and mapping (SLAM) problem.
Fig. 1. 5G SLAM scenario with the propagation environment and two vehicles.
Fig. 1 shows the 5G SLAM scenario, where 5G communication links can be used to share measurements, map, or location information, leading to cooperative positioning and mapping. In 5G mmWave cooperative positioning and mapping (i.e., positioning and mapping based on measurements from 5G mmWave communication signals), there are three main tasks: (i) Vehicle positioning: determine the states (position, velocity, heading, clock bias) of the vehicles; (ii) Environment mapping: estimate the number of objects, as well as each object’s type and position; and (iii) Cooperation: in the BS fusing the collected the map information from the vehicles, and relay it to each vehicle.

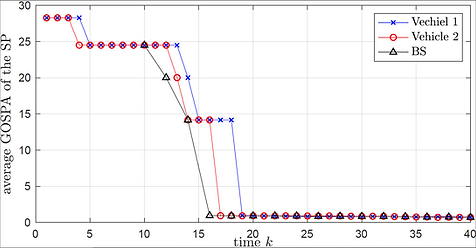
Fig. 2. Mapping accuracy of 5G SLAM without cooperation (left) and with cooperation (right).
Fig. 2 reports the mapping accuracy, represented by the GOSPA results. In Fig. 2a, we see that the GOSPA per vehicle goes down as they move in the environment. The GOSPA at the BS is reduced faster, as it can benefit from the information of all vehicles. In Fig. 2b, we note that when the BS sends back the map to the vehicles over the downlink, each vehicle can benefit from the measurements of the other vehicle, so that the GOSPA is reduced faster on the vehicle maps as well. Video represents 5G SLAM.
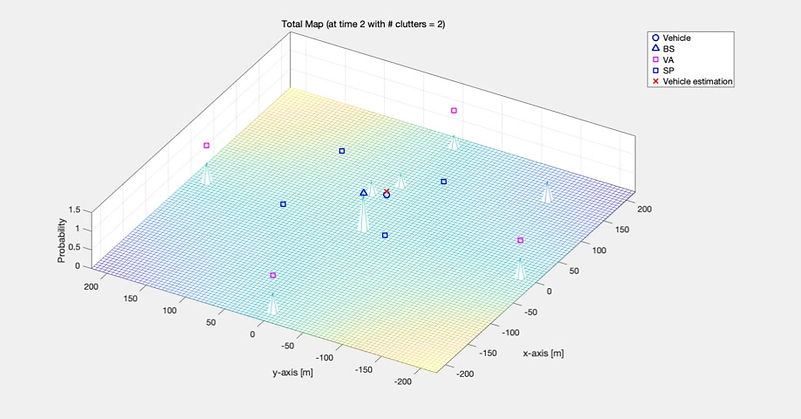
One of Integrated sensing and communication's (ISAC's) most essential uses, SLAM, is gaining traction. SLAM is a technique that obtains environmental information of an environment without previous knowledge and simultaneously locates a user; as it needs a huge volume of various types of high-precision data measurement, it is necessary to implement ISAC based on mmWave and THz [1, 2]. As shown in Fig. 3, we propose a SLAM method that maps arbitrary-shaped objects using mmWave/THz-based radio SLAM and achieves high-precision user location. The proposed SLAM technique detects the types of a number of objects in the radio wave environment, classifies each object to perform environment mapping, and utilizes this mapping information to improve the positioning accuracy of the user.
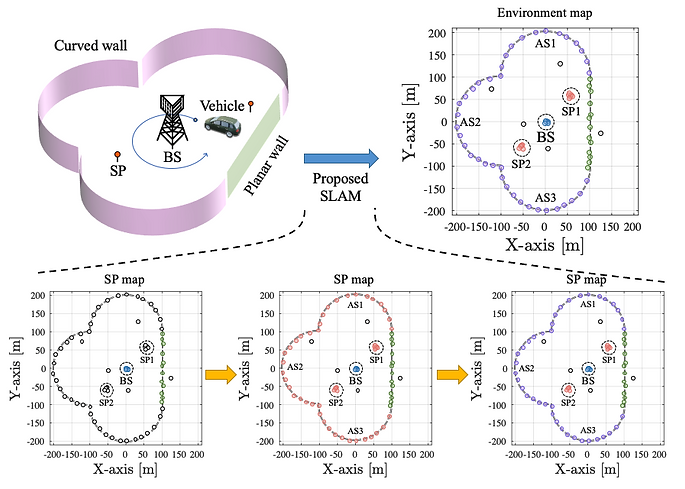
Fig. 3. An illustration of the 6G SLAM
[Related publication]
-
H. Kim, K. Granström, L. Gao, G. Battistelli, S. Kim and H. Wymeersch, “5G mmWave Cooperative Positioning and Mapping using Multi-Model PHD Filter and Map Fusion,” IEEE Trans. Wireless Commun., vol. 19, no. 6, pp. 3782-3795, Jun. 2020.
-
J. Lee, H. Kim, H. Wymeersch, and S. Kim, "Dirichlet process approach for radio-based simultaneous localization and mapping," 2021, arXiv:2107.00864. [Online]. Available: https://arxiv.org/abs/2107.00864
2. Sensing and Communication time optimization
Integrated sensing and communication (ISAC) is a new paradigm in which previously competing for sensing and communication tasks can be jointly optimized. In future 6G systems, higher-frequency(mmWave to THz), wider bandwidths, and denser large-scale antenna arrays provide an opportunity to integrate wireless signal sensing and communication into a single system and the entire communication system to act as a sensor. Context information (localization, imaging, environment reconstruction, etc) obtained from sense can help improve communication performance, such as high-precision beamforming and less overhead channel state information (CSI) acquisition.
In the ISAC system, reducing or optimizing bi-static sensing/channel estimation overhead is a critical challenge to improve the data rate in mmWave/THz communication [1]. As shown in Fig. 4, we propose a scheme optimizing the per-user channel sensing duration in multi-user multiple-input single-output (MU-MISO) systems. For each user, the BS predicts the effective rate to be achieved after pilot transmission. Then, the channel sensing duration of each user is optimized by ending the pilot transmission when the predicted rate is lower than the current rate.

Fig. 4. An illustration of the optimizing channel sensing duration in the ISAC system
[Related publication]
-
J. Kang, H. Wymeersch, C. Fischione, G. Seco-Grandos, and S. Kim, “Optimized Switching Between Sensing and Communication for mmWave MU-MISO Systems,” accepted to 2022 IEEE Inter. Conf. Commun. Workshops (ICC Workshops), Mar. 2022.
bottom of page