
Channel Sensing and Tracking
1. mmWave Beam Tracking (Beam Mangement)
Beam alignment is required in millimeter-wave communication to ensure high data rate transmission. However, with narrow beamwidth in massive MIMO, beam alignment could be computationally intensive due to the large number of beam pairs to be measured. To target this problem, we’ve studied the location-aware beam alignment framework by exploiting the location information of the user equipment (UE) and potential reflecting points [1]. Both UE and BS perform a coordinated beam search from a small set of beams within the error boundary of the location information. The selected beams are then used to guide the search for future beams. To further reduce the number of beams to be searched, we propose an intelligent search scheme within a small window of beams to determine the direction of the actual beam. The BS and UE coordinate the search iteratively and the search is stopped when the target rate is achieved.
In mmWave mobile systems, the beam tracking is required to maintain the beam alignment. Two common tracking methods for mmWave beamforming are codebook-based method and perturbation method. However, the codebook method is limited by the resolution of the codebook whereas perturbation method requires lengthy beam training which incurs large overhead as well as delay, rendering these approaches unsuitable for mobile environment. To solve this problem, we’ve studied the filter-based beam tracking which estimates the channel parameters (channel gain and angle) and error compensation method which control the beamwidth adaptively. In [2], the BS tracks the UE based on particle filter and, the adequate beamwidth is set via the partial activation of the antenna array. In [3], the channel is tracked with the extended Kalman filter (EKF) at the BS and the beamforming weight is updated with a robust minimum mean squared error beamformer bounded by the array vector error which is fed from the error variance estimated by the EKF.
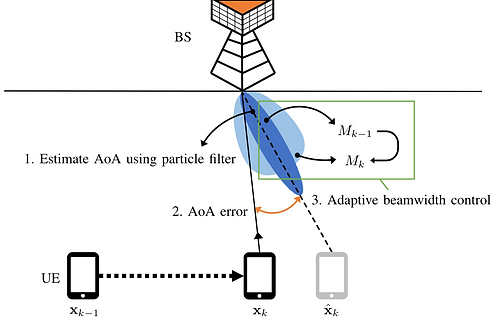
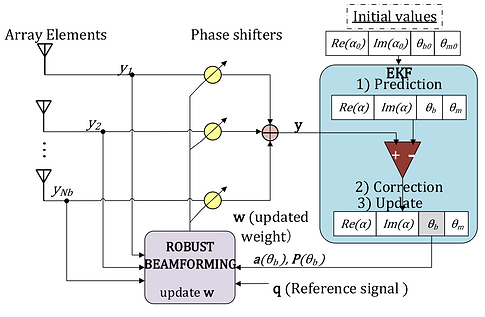
[1] Orikumhi, J. Kang, C. Park, J. Yang and S. Kim, "Location-Aware Coordinated Beam Alignment in mmWave Communication," in Proc. IEEE Allerton 2018, Monticello, USA, Oct. 2018.
[2] H. Chung, J. Kang, H. Kim, Y. M. Park, and S. Kim, “Adaptive Beamwidth Control for mmWave Beam Tracking,” accepted to IEEE Commun. Lett., Sep. 2020.
[3] S. Jayaprakasam, X. Ma, J.W. Choi, and S. Kim. “Robust Beam-tracking for mmWave Mobile Communications,” IEEE Commun. Lett., vol. 21, no. 12, pp. 2654-2657, Dec. 2017.
2. UAV Tracking
Unmanned aerial vehicle (UAV) is widely used for military, delivery, and disaster because of many advantages of network formation, high mobility, and low cost. It is expected that the UAV control technique for target tracking is essential because UAV collects environmental knowledge and provides an effective wireless network to ground users. We have been studying deep reinforcement learning (DRL) based on multiple UAV control for target (i.e., ground user) tracking. DRL is an emerging tool to select optimal action in a dynamic system, for example, autonomous driving, UAV control problem and wireless network, etc. By applying DRL to multiple UAVs system, UAVs learn how to take actions to track/localize the ground target in a complex 3D environment. Besides, the DRL-based UAV control technique mitigates computation time that becomes critical when the number of UAVs or targets increases.
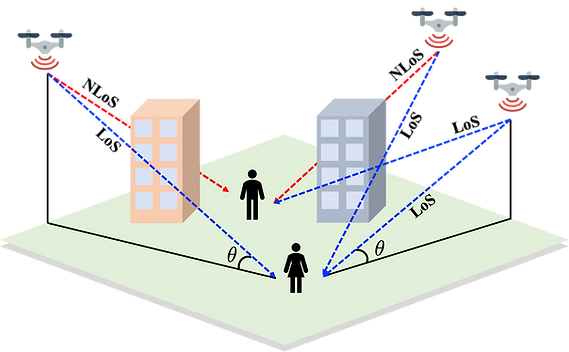
Fig 1. Multiple UAVs track ground users in challenging 3D environment
Multiple UAVs are assigned to tracking missions in the existence of multiple ground targets. Each of the UAVs is equipped with a range sensor that measures the distance between UAVs and targets. The measurements received by UAVs are blocked due to obstacles and structure.
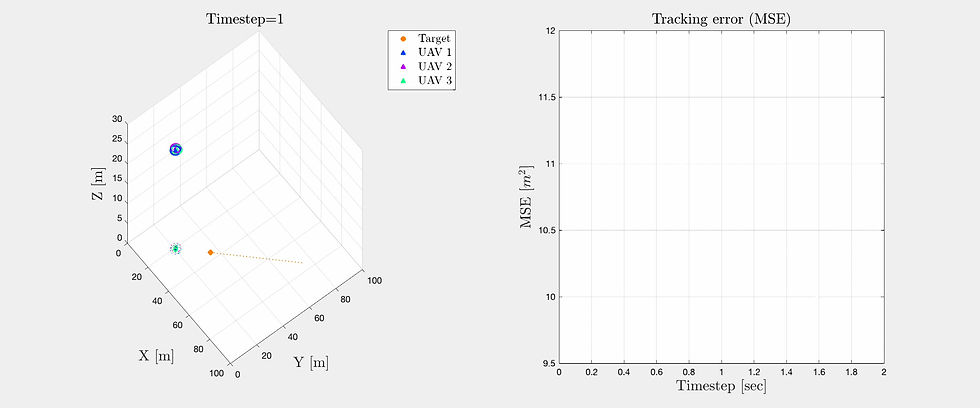
Video1. The results of 3D trajectories of UAV and target (left), and localization error (right) during the tracking missions
While operating tracking missions, UAV’s flight direction is determined by the trained-DRL network. Tracking error measures MSE between the true target position and estimated target position. Applying DRL to target tracking enables UAVs to track and localize ground users accurately.
3. Direction Finding
Direction finding is an essential technology to counter enemy air infiltration and threats and is also widely used in array signal processing such as radar, sonar, and wireless communication systems. Direction of arrival (DoA) estimation using an array antenna to find the direction of unknown signals is a representative direction finding method.
During the last decades, subspace-based DoA estimation algorithms like multiple signal classification (MUSIC) and estimation of signal parameters via rotational invariance techniques (ESPRIT) were proposed. Also, with the rapid development of artificial intelligence (AI) technology, many machine learning-based DoA estimation algorithms were proposed.
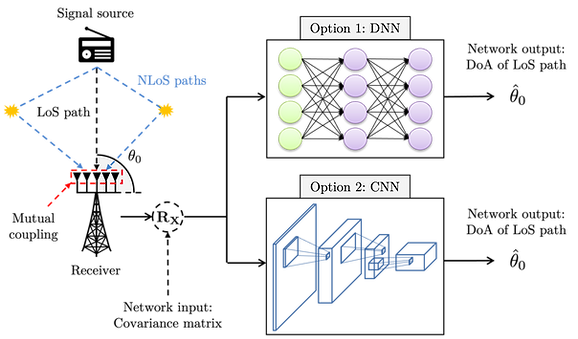
Fig 2. A scheme of deep learning-based DoA estimation.
Our lab mainly focuses on various machine learning-based DoA estimation for practical applications. We are also using the testbed for real-world data collection and DoA estimation algorithm validation.

Fig 3. A picture of transmitter and receiver used for the experiment.